für Recht und Ökonomik
Selected Publications: Deep Learning and Financial Stability
7. Januar 2021, von Pedro Magalhães Batista
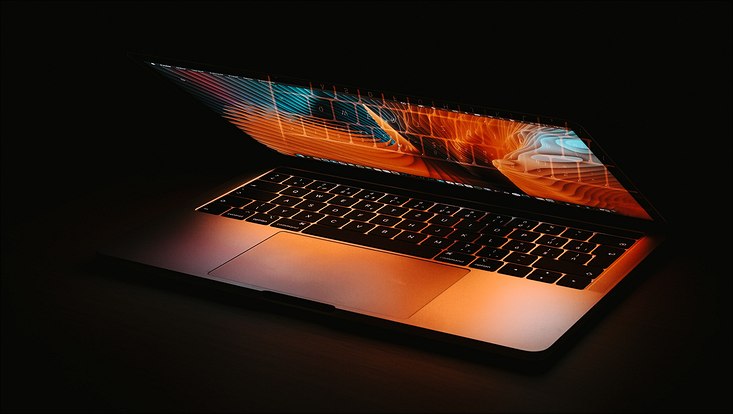
Foto: JanVlacuna
Gary Gensler and Lily Bailey have a new working paper, "Deep Learning and Financial Stability." Deep learning represents a discontinuity from prior analytical tools. It is already used for regulatory compliance and market surveillance, but it is also starting to be implemented for trading and asset management, and risk management and underwriting. Coupled with natural language processing, its utility is further extended. However, the authors point that beyond its efficiency gains and higher financial inclusion, deep learning may lead to increased systemic risk after broader adoption. The paper starts by bringing us the key characteristics that turn deep learning a unique development. Then, they present us how such development interacts with systemic risk - how the failure of one node may propagate negatively through the network.
According to the authors, systemic risk occurs especially through three channels, monocultures, network interconnectedness, and regulatory gaps. And deep learning can accentuate these limitations through five possible pathways, data, model design, regulation, algorithmic coordination, and user interference. Finally, the authors suggest that to mitigate these challenges, we need to rethink the financial system and a mix of micro- and macro-prudential policies are required. Among micro policies, they suggest internal mapping of institution-wide dependencies on data, model hygiene, firm buffers, and regulatory diversity - regulators, to address the tradeoff between the need of explainability and the incentives towards uniformity, should propose multiple ways to internalize regulations. Among the macro policies, external mapping - such as stress testing -, material external dependencies, horizontal reviews, network buffers, and ex-post interventions should be considered.